ML
Machine Learning in Banking: Is it Worth Implementing ML to Your Software?
What is modern ML, and how does it affect help FinTech businesses and banks worldwide to be a step further than competitors?
Overall, machine learning isn't a new concept, but it's taken a quantum leap thanks to the big data revolution and the cost of computing power. These developments have demonstrated the true potential of ML in multiple industries, including finance, medicine, and jurisprudence.
Machine learning in banking and financial sectors is used for automated decision-making systems. Employed in online Automated Decision-Making (ADM) tests, the system determines the probability of a potential borrower to fulfill their loan commitments.
Wanna dive in into world of real-time machine learning and its impact on data analysis? Keep reading to learn why you should adopt AI and machine learning in banking, and examine real-world machine learning in banking examples.
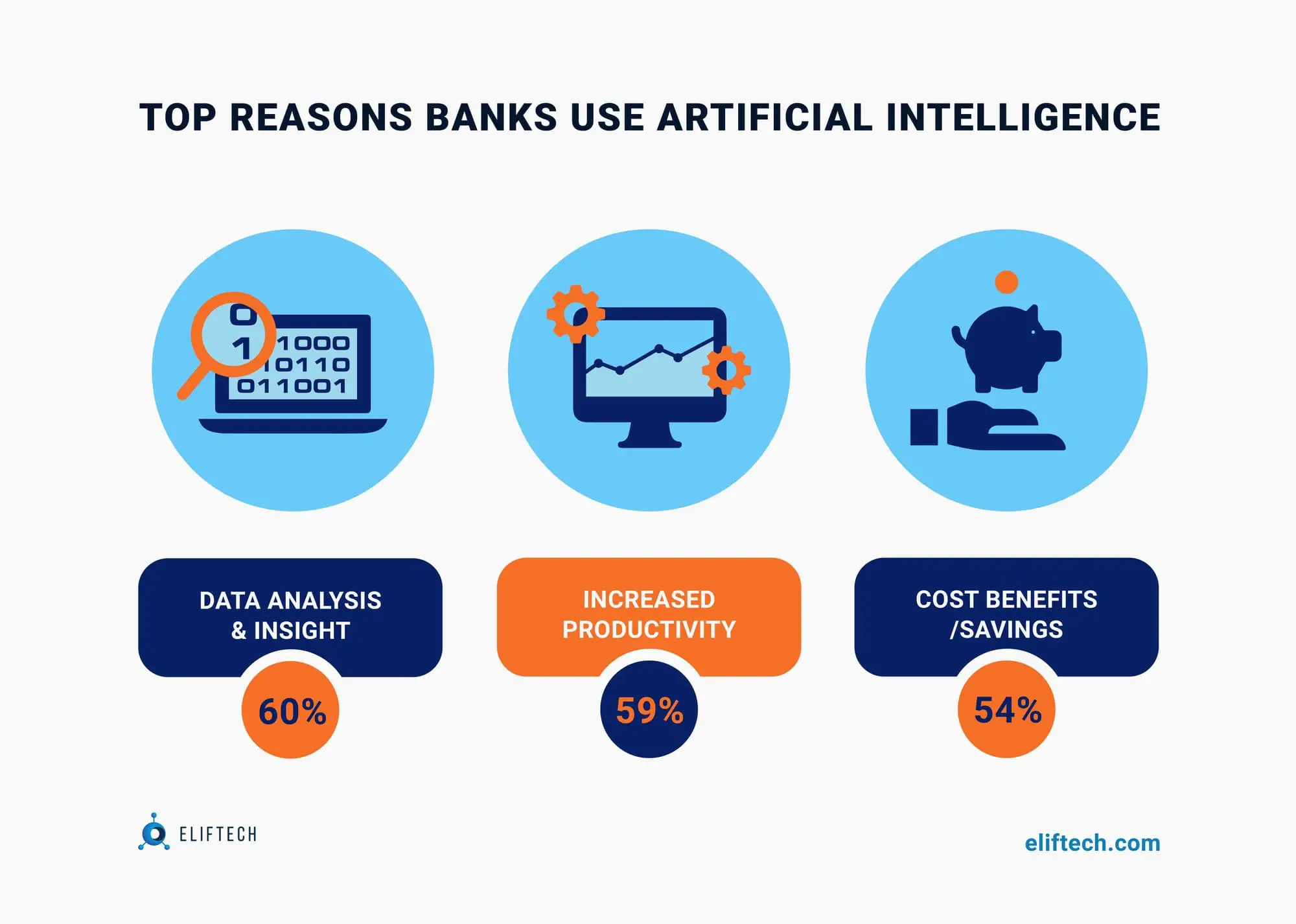
Machine Learning in Banking: Definition, Impact, and Role in the FinTech Landscape
Over the past twenty years, machine learning has seen a big surge in popularity, thanks to powerful computers, the Internet, and the digitalization of information. The term has been used in science for over half a century to describe programmed pattern recognition. However, the concept is even older. Mathematicians started talking about such processes at the beginning of the 19th century.
McKinsey's analysts have taken a close look at how ML algorithms are used in business, especially in banking. They found that the idea of "perfect intelligence" has somewhat distorted our understanding of what machine learning is and what it can truly offer.
So, what exactly do we mean by Machine Learning today?
Machine learning (ML) in artificial intelligence refers to the decision-making process with the help of a computer based on a statistical algorithm. This technology finds its application in various areas, in particular, banking to predict product recommendations or decid whether to approve a loan.
One of the main ML advantages is that it makes decisions faster and cheaper than humans in automated business processes. It's also believed to enhance decision quality by eliminating human biases.
Battling Bias in Machine Learning (three-level approach)
However, one challenge in the world of machine learning is bias. Like humans, these AI algorithms can sometimes make unfair decisions. For example, if a borrower has always paid their loans on time, they might be considered a low-risk borrower. But what if they had tax benefits that made it easier for them to do so? The algorithm might not know about these benefits, and that could lead to a wrong decision.
But here's the good news: we can fight bias in machine learning. We've got a three-level plan:
Level 1: Users of ML algorithms need to understand their limitations. It's like driving a car – you can't just push a button and expect it to work perfectly. You need to know how it operates.
Level 2: Data scientists, responsible for these algorithms, can help by shaping the data they use to train the algorithms. But here's the tricky part: sometimes, the data we have isn't enough. We need fresh, unbiased data to make things right.
Level 3: Business leaders play a crucial role too. They need to decide when to use machine learning and when not to. It's all about finding the right balance between convenience and transparency.
Don’t Ignore the Humans: The Value of User Experience
Let's talk about how users see machine learning algorithms. To most users, these algorithms are like black boxes – they provide quick and easy solutions without revealing what's happening inside. Users often rely on them without much understanding of their inner workings.
But here's the thing, good judgment should be based on knowledge. Business users who want to make sure they're not using algorithms in harmful ways are a bit like consumers who want to eat healthy food. Just as health-conscious consumers read nutrition labels to avoid excess calories or harmful additives, users should take a closer look at the algorithms they're using for their business.
For example, when these algorithms assess someone's creditworthiness, they sometimes have a built-in bias that prevents them from considering rapid changes in a loan applicant's behavior. One key factor here is the length of the credit term. Customers who are considered higher risk tend to opt for longer-term loans, anticipating possible difficulties in repaying them.
On the other hand, lower-risk customers often choose shorter-term loans to minimize interest costs. A machine learning algorithm might pick up on this pattern and assign higher risk ratings to longer-term loan applications. But what if a client, in an attempt to avoid a high-risk rating, chooses a shorter-term loan and then can't meet the monthly payments due to the higher amount? In such cases, the system might not react quickly, leading to increased credit losses.
So, when a business is thinking about using an algorithm to solve a problem, it needs to find a balance between the costs and benefits. To choose the needed machine learning algorithm, it's essential to consider a few key questions:
- How quickly do we need a solution? Time is often critical in solving business problems. An optimal statistical model can become outdated before it's even completed. In rapidly changing business environments, a machine learning algorithm developed overnight can outperform traditional models that take months to build. That's why machine learning algorithms are well-suited for tackling issues like fraud.
- What data do we have to work with? Algorithms rely on data. If the data available is insufficient, it might be a good idea to bring in a consultant who can help the organization gather the necessary information.
- What problems should be solved? One of the great things about machine learning is that it can tackle problems that were either unnoticed before or seemed too costly to solve using traditional methods. When dealing with these kinds of challenges, organizations should pinpoint the ones that have a substantial impact on their business and bring in skilled data processing experts to handle them.
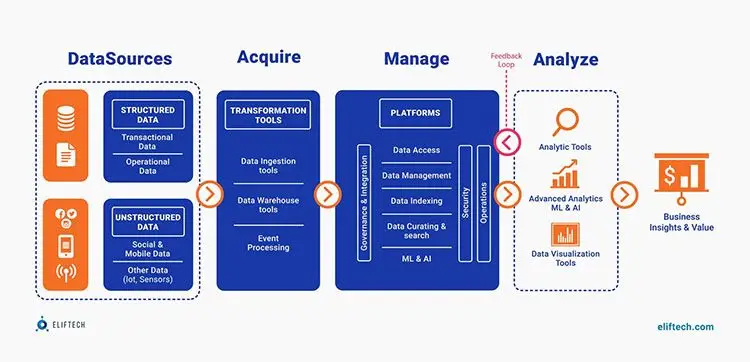
Embracing Machine Learning in Banking & Other Industries
Businesses can and should embrace machine learning without getting overwhelmed by risks. Here's how they can do it:
- Step 1: Establishing Business Standards
The first step is to establish clear business standards for validating machine learning models. This means creating a template for documenting these models, standardizing the acceptance process, and including essential information like the business context. It's crucial to ask specific questions about how the model will impact the business, what data it will use, and what tradeoffs there are in terms of cost and expenses.
Importantly, this process needs active participation from users to find the most suitable solution for the business problem. Passive checklists or guidelines often don't cut it. You'll also need to define key parameters for the model, including the standard analyses to be performed on input data, processed samples, and simulation results. Lastly, you discuss the model with business users to ensure alignment.
- Step 2: Professional Verification
Next up, you should establish a clear and rigorous process for verifying and approving ML algorithms. The level of scrutiny may vary depending on the industry and business context, especially in terms of potential economic consequences. The process should set review standards and create an ongoing program to monitor new models.
Consider the unique characteristics of machine learning models, such as automatic algorithm updates when new data becomes available. If your algorithms receive regular updates, like weekly changes, make sure validation procedures happen within hours or days, not weeks or months.
- Step 3: Continuous Knowledge Development
To successfully embrace machine learning, banks and other financial entities must invest in the development and sharing of knowledge in both information technology and business applications. Stay vigilant by learning new ideas and best practices of application of machine learning in banking. This ongoing effort fosters a culture of constant knowledge improvement and expertise in the field.
Why Adopt Machine Learning in Your FinTec Business?
Machine learning can bring many benefits not only to large companies but to almost any organization, regardless of its size and direction of activity.
With more intelligent algorithms adopted by nearly every industry today, let's see how exactly ML can enhance your business (in particular, machine learning in banking and finance):
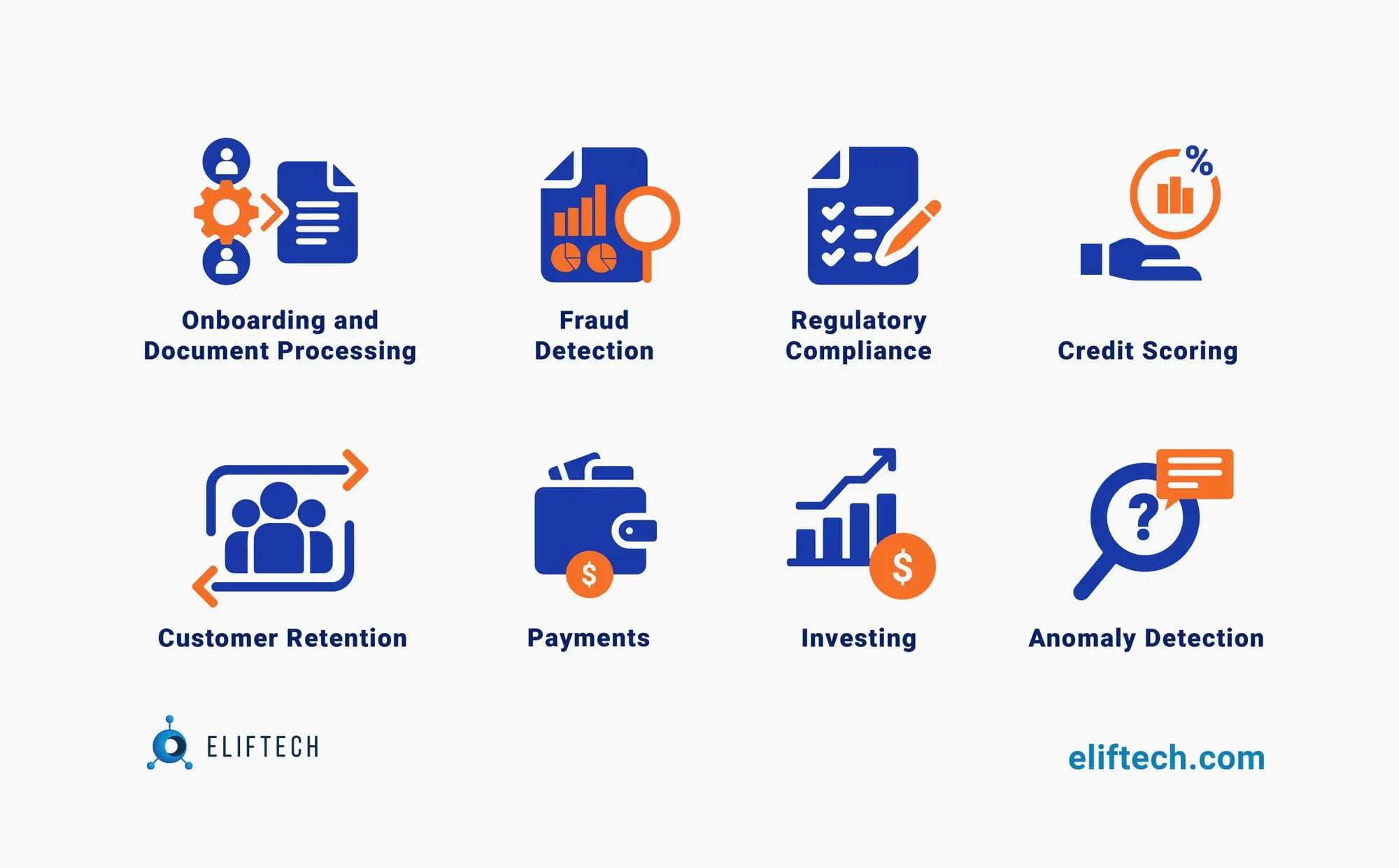
- Processing vast amounts of data: ML can handle massive volumes of data that would be overwhelming for traditional methods. Small businesses can use ML to make informed decisions about product development, customer attraction, and internal processes.
- Automation of routine tasks: Automation is a core function of ML. It saves time, enhances resource management, and reduces expenses. ML can automate tasks like data classification, report creation, threat monitoring, abuse prevention, and internal audits.
- Enhancing marketing personalization: ML empowers personalized marketing by creating tailored campaigns for countless users. Even small businesses can benefit through digital platforms like Facebook and Google, which integrate ML for microtargeting campaigns.
- Spotting business trends: ML excels at identifying trends within large datasets that may elude human recognition. Small and medium-sized businesses use ML to predict customer churn, improve hiring processes, and make informed decisions.
- Accelerating development cycles: ML acts as a super-intelligent assistant in research and development, reducing the need for trial and error. This frees up time for innovation, but its implementation may require structural changes and adjustments.
- Enhancing cybersecurity: In an era of increased cyber threats, ML is a valuable tool for safeguarding debit card payments. It works best when integrated into a layered security approach, including location tracking, to detect suspicious transactions.
Better Safe than Sorry: What about Security?
During the pandemic, many people turned to debit payments due to financial uncertainties caused by lockdowns, which made them wary of accumulating credit card debt. According to a recent study, around $100 billion in annual credit card spending is expected to shift to debit cards.
Debit payments are based on the money already available in the user's bank account, providing a sense of security. However, there's a risk of scammers stealing debit card details, even though such incidents account for less than 1% of card purchases. Victims of debit card scams often endure lengthy and stressful procedures to recover their money.
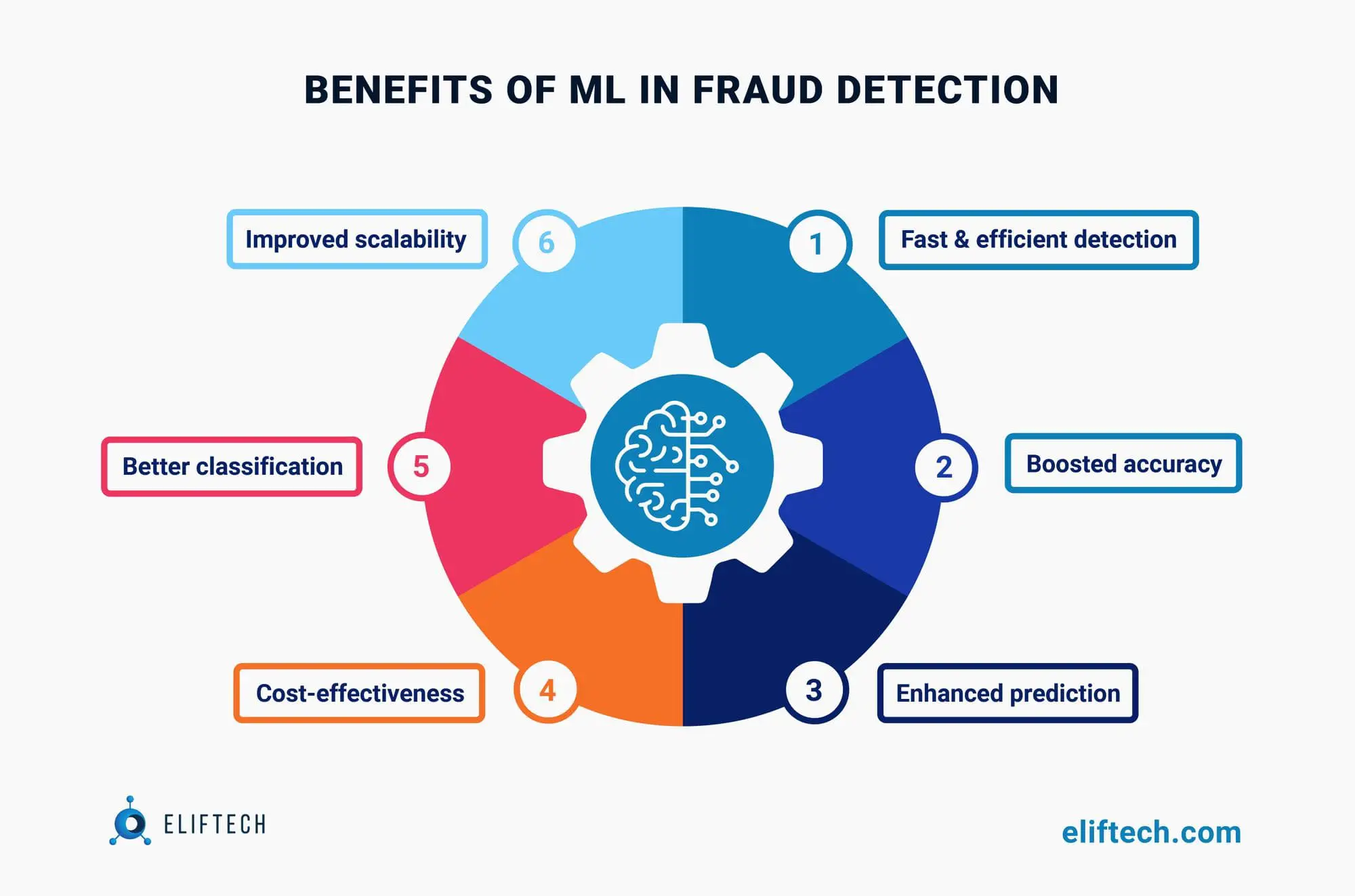
Machine learning plays a crucial role in combating cybercriminals attempting to compromise debit payments, especially those involving international fraudsters. Yet, experts at People's United Bank suggest that the best approach involves a layered security strategy. Additionally, location tracking helps financial institutions identify suspicious transactions, such as when a user makes a mobile payment from a city different from their usual residence or registration. However, implementing this security measure can make it more challenging to attract new customers.
The pandemic and quarantine have accelerated payment habits toward cashless payments. As a result, users are more actively switching to non-cash payments and more often using e-commerce services. At the same time, the growing popularity of contactless payment instruments and settlements with them continues.
Top 5 Machine Learning Use Cases in Banking
Leading U.S. banks are at the forefront of innovation when it comes to safety and efficiency, and they are investing significantly in machine learning and banking technologies.
In fact, ML has become a key driver of success in the banking industry, as evidenced by the top five U.S. banks. The U.S. Federal Reserve recently published an official report covering the state of the U.S. banking system, focusing on financial institutions with capital exceeding $300 million.
Unsurprisingly, the top five banks on this list have made substantial investments in integrating artificial intelligence and machine learning into their services and offerings
- JPMorgan Chase
- Wells Fargo
- Bank of America
- Citibank
- U.S. bank
These banks employ ML in various areas, ranging from sorting emails using NLP algorithms to automatically updating information in customer relationship management (CRM) systems. They also provide more convenient mobile banking services with the assistance of ML. For instance, these banks use machine learning models to predict stock price movements and manage investments and stock trading better.
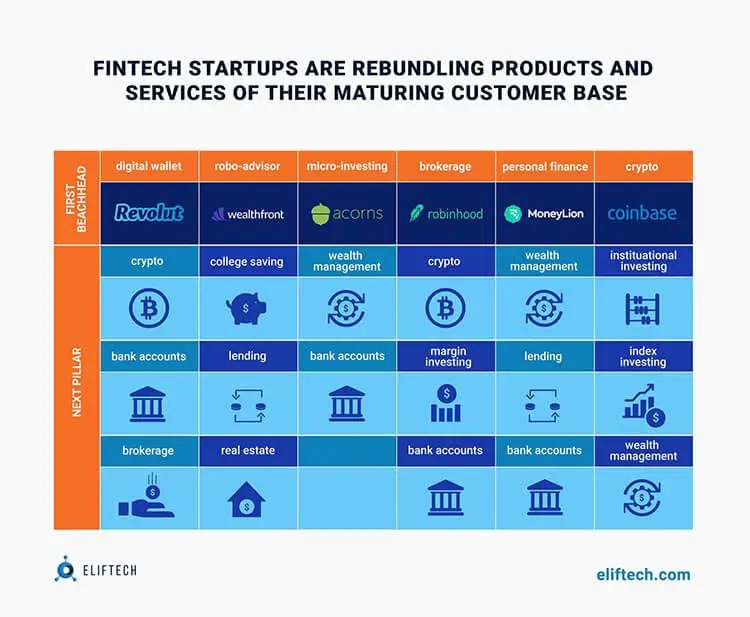
In 2022, these top five banks closed 400 local branches and shifted customers to ML-powered mobile banking. Surprisingly, this move not only avoided profit loss but also exceeded profitability goals, drawing in many new customers. The key to this success? ML-enhanced offerings exceeded customer expectations, generating positive word-of-mouth marketing.
- JPMorgan Chase and COiN
JPMorgan Chase employs the Contract Intelligence (COiN) ML algorithm to quickly process loan agreements, saving thousands of hours. The bank is exploring new applications for this tool to enhance efficiency and profitability. Additionally, their Emerging Opportunities Engine identifies potential customers for additional services, initially in equity trading, and now expanding into debt capital trading. A virtual chat assistant is also being piloted to streamline customer support.
- Bank of America and Erica
Bank of America introduced Erica, a virtual assistant using cognitive analysis and predictive analytics. With over 45 million users, it aims to simplify routine transactions and improve customer support, resulting in substantial growth and investment in technology.
- Wells Fargo and ML Chatbots
Wells Fargo's AI & ML Enterprise Solutions team launched an ML chatbot on Facebook Messenger, simplifying tasks like password resets and account inquiries. This feature, tested by 700 employees, will be available to all customers.
- Citibank and FeedzAI
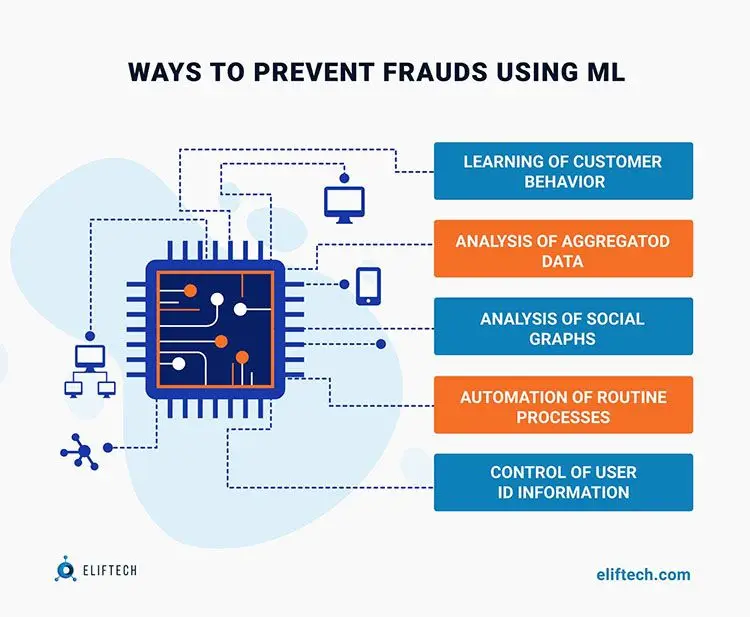
Citibank invested significantly in FeedzAI, a global enterprise specializing in using machine learning to detect and prevent fraudulent transactions. FeedzAI analyzes vast amounts of data in real-time, alerting financial institutions to potential fraud cases.
U.S. Bank's ML Initiatives
U.S. Bank embraces technology through its ML Innovation Leader group, focusing on conversational interfaces and chatbots to enhance customer service. They aim to use machine learning to help employees respond more efficiently to customer queries, emphasizing that technology complements human efforts rather than replacing them.
Machine Learning for Banking: Key Considerations
According to a study by the World Economic Forum and Deloitte, 76% of banking executives view artificial intelligence as a top priority. ML, once limited to budgeting and digital tools, now plays diverse roles in areas such as payment segmentation, personalized customer suggestions, and chatbot-driven customer interactions — illustrating the benefits of machine learning in banking.
Substantial Cost Savings
The potential for banks to save $447 billion by 2023 through adoption of machine learning for banking industry, with $416 billion from front and middle office optimizations. AI-driven biometrics and personalized offerings reduce customer interaction times, while ML mitigates risks associated with dubious clients engaging in money laundering.
Industry-Wide Recognition
An OpenText survey reveals that 80% of banks recognize the value of machine learning, actively utilizing it in chatbots and fraud prevention.
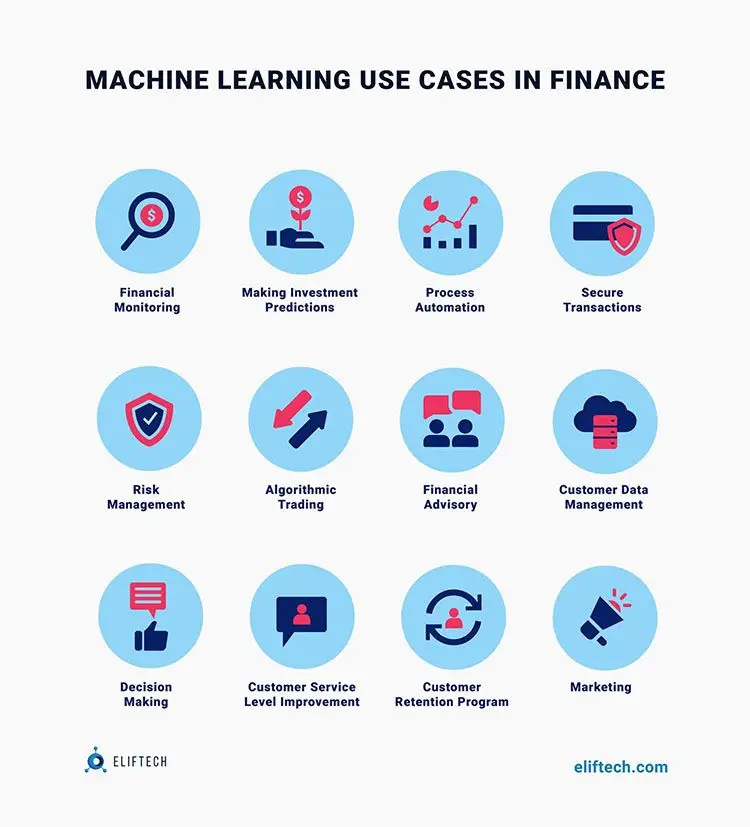
Security Concerns
U.S. Bank employs AI to enhance the customer experience, combat fraud, and make lending decisions. Voice assistants like Google Assistant, Siri, and Alexa provide consultations. Deep machine learning identifies suspicious patterns, reducing fraud and ensuring cybersecurity.
Personalized Offers
Citibank leverages ML to seamlessly engage with customers through real-time data analysis. The platform creates timely personalized offers and comprehensive customer profiles, improving service quality and efficiency.
Elevating User Experience
Machine learning fosters customer loyalty by helping banks, like U.K. startups Trussle and Habito, develop tailored financial products. Account managers, equipped with ML tools, can guide clients more effectively. They can also analyze customer experience with banking services through existing channels. This allows banks to determine how well their current processes are performing, such as any bottlenecks.
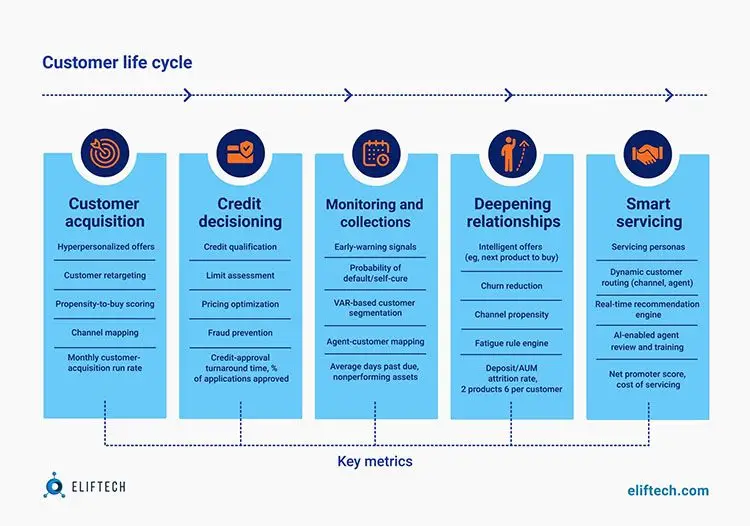
Process Optimization
Machine learning streamlines operations and enhances the customer experience. Banks use it to verify tax payments during payroll processing, speeding up fund transfers.
Empowering Human Potential
ML frees employees from repetitive tasks, allowing them to focus on more meaningful intellectual work. This shift positively impacts operational processes and internal Net Promoter Scores (NPS), enhancing overall efficiency.
Machine Learning in Banking: Is It Worth It?
As you can see, the top five U.S. banks have all invested heavily in ML. They focus on providing the next level of customer self-service, fast document processing, using chats, fraud checking, loan portfolio management, etc. All of them have already reaped the rewards, demonstrating remarkable earnings growth.
To summarize, how does machine learning work for a financial entity? Based on the experience of leading U.S. machine learning banking use cases, these are the top benefits of AI and ML in banking:
- Personalization: Tailoring marketing offers to individual customers across various channels ensures that your message resonates and drives conversions.
- Precise consumer targeting: Leveraging big data within your CRM helps gain deep insights into customer needs. By analyzing both internal and external data, you can create comprehensive customer profiles and deliver precisely targeted services.
- Enhanced user experience (UX): ML algorithms can assess factors like a user's device, location, browsing history, and past interactions to provide a personalized UX. This not only boosts satisfaction but also translates into increased sales.
- Self-service portals and chatbots: Given the preference for self-service among millennials, investing in web portals and mobile apps with ML-driven chatbots is a strategic move. In fact, 44% of U.S. citizens favor chatbot interactions over traditional customer support methods, making it a valuable avenue for future growth.
What's more, the above-mentioned ml use cases in banking clearly indicate that the top five U.S. banks are taking ML very seriously. Their growth indicates the importance of ML solutions implementation to existing banking software.
But can other less-known banks effectively compete with the industry giants? Absolutely YES, even despite the significantly lower budget. The access to machine learning and ML-infused platforms is getting cheaper yearly. After all, the question isn't just "who invested more?" but rather "who invested better?"
Looking for a hands-on team to implement your banking system with ML algorithms? ElifTEchh can help you implement machine learning solutions that can give a competitive edge in the FinTech industry. Contact our AI and ML experts for a free consultation.
FAQ
What are the challenges of machine learning in banking sector?
Machine learning in banking faces challenges such as data privacy concerns, ensuring model transparency and fairness, and dealing with regulatory compliance. Additionally, acquiring and managing high-quality data can be a hurdle in building effective banking machine learning for financial institutions.
How is ML transforming the banking industry?
Machine learning banking enables automated decision-making, personalizing customer experiences, and streamlining operations. It helps banks make data-driven lending decisions, deliver tailored product recommendations, and enhance cybersecurity. ML also empowers banks to optimize processes, reduce costs, and stay competitive in the digital era.
Browse our case studies and get actionable insights to drive your success
See more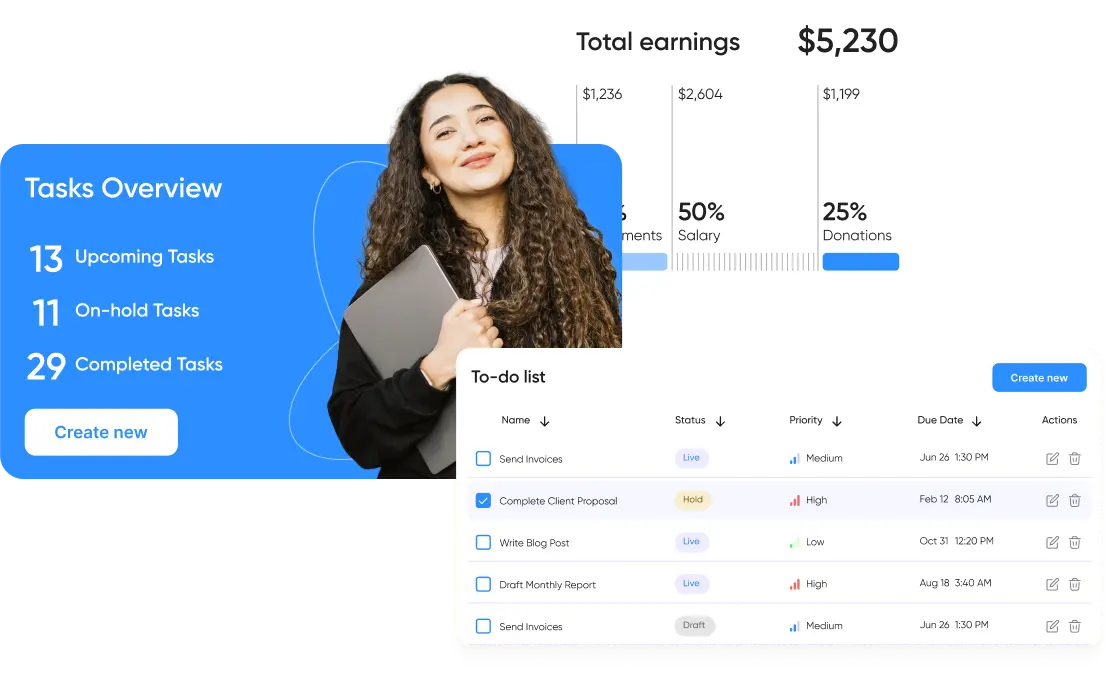